Dataiku supports all kinds of users, whether they prefer to leverage the visual point-and-click interface or work entirely in code. But just because Dataiku has a simple-to-use graphical user interface doesn't mean we've skimped out on robust features for more technical profiles. This blog post will detail a few of the highlights Dataiku has to offer for data scientists, engineers, architects, and other profiles who may prefer to work with code — rather than visual tools — to manipulate, transform, and model data.
Use Your Favorite Languages and Tools
Dataiku allows you to work with the tools you already know and love without sacrificing collaboration with other team members, who may or may not be using the same set of tools.
For example:
- Create code recipes in the language of your choice, including Python, R, SQL, and more.
- When developing code directly in Dataiku, use the native code editor or opt for a more familiar experience with embedded Jupyter Notebooks or Code Studios for popular web-based IDES like VS Code, R Studio, or Jupyter Labs.
- If you already have Jupyter Notebooks that have been developed outside of Dataiku, you can upload those Notebooks manually or connect to a remote Git repository and use the typical branching, push, and pull actions to keep your code in Dataiku synced with that remote repository.
- Dataiku includes built-in algorithms from state-of-the-art machine learning libraries, such as Scikit-Learn, MLlib, and XGboost, plus TensorFlow and Keras for deep learning. But, you can also code your own custom models and still take advantage of all the benefits Dataiku Visual ML has to offer, such as automatic experiment tracking and diagnostics, interpretability and performance metrics, auto-documentation, and ease of version monitoring in production.
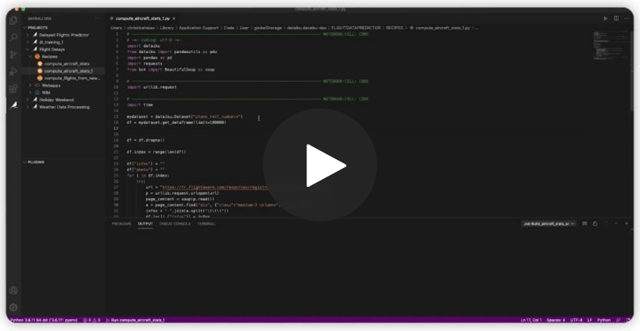
When it comes to model deployment, with just a couple clicks, you can deploy a model or other functions as a RESTful API service to incorporate its outputs into other data pipelines, visualizations, or applications. Thanks to bi-directional integration with MLflow, Databricks, and cloud ML platforms such as AWS Sagemaker, AzureML, or Google Vertex AI, you can design and experiment in one place and deploy & monitor in another. In short, you’re not bound by the limitations of a single platform; you have the freedom to choose the best tools for your specific needs while enjoying the centralized standardization, explainability, and AI Governance that Dataiku offers.
Easily Reuse & Share Code
Easy reuse and sharing of code, datasets, and other assets in Dataiku helps teams reduce inefficiencies and inconsistent data handling while simultaneously empowering less technical users to go further on their own.
Dataiku project and global libraries are a great way teams can centralize and share code both within and across projects. And while Dataiku comes pre-loaded with starter code for many common tasks, you can easily add your own code snippets for you and your team to use. To aid in upskilling and improve speed to value for code-first profiles, the Dataiku Developer Guide contains countless tutorials and articles — everything from the Dataiku API reference documentation to information on creating applications in different frameworks, performing security and resource-management admin tasks, and operating Dataiku programmatically.
Goodbye, Complexity; Hello, Efficiency
Dataiku abstracts away and simplifies layers of complexity related to connecting to data and configuring compute resources. For example, data scientists can seamlessly execute their code in a containerized, distributed way using Spark or Kubernetes clusters — simply select the runtime environment you want, and Dataiku takes care of spinning up the containers and shutting them down when the job is done. In other words: spend more time doing what you love, and less time troubleshooting resource and environment issues and Spark configurations!
Go Faster With Generative AI
Speaking of complexity, large language models (LLMs) may arguably be the most exciting (but most complicated) technology to hit the data science landscape in the past few decades. With dozens of models available through paid service providers and open source platforms, it can be hard to know how to safely apply and scale this new tech to solve enterprise problems. Dataiku’s LLM Mesh provides a powerful backbone for generative AI applications that addresses your company’s concerns about cost management, compliance, privacy, and technological dependencies. As an application developer, you’ll also appreciate Dataiku’s built-in Prompt Studios, RAG components, and AI Code Assistants that help you maximize your effectiveness and speed to value.
To wrap it up, Dataiku’s platform is like an AI toolbox designed for both coders and non-coders — it's got everything from nifty shortcut gadgets to powerful engines to safety equipment. For data engineers and data scientists, it's not just a sandbox for prototyping; it's a production-grade workshop where you can dive in, develop and test your code, and bring your data projects to life.