Most data-conscious people know that building an AI-enabled data operation inside one’s company is a challenge with many obstacles. From upskilling data workers and business analysts, to defining objectives and processes, and to extracting value, the task is enormous and complex. At the same time, achieving Everyday AI is not a destination that is reached all at once, but a journey dotted with the milestones of incremental, meaningful victories.
Over the past decade and more, the path that defines the journey to Everyday AI has run through a data industry landscape that is increasingly defined by its focus on intelligent model regulation and data privacy controls — in brief, on Responsible AI. The focus of the industry has turned, for this reason, toward AI Governance, a field in which Dataiku excels.
Governance, regulation, and data privacy were hot topics at this year’s Everyday AI conferences in New York, London, and Bengaluru. In this blog, we’ll take a look at the key insights and takeaways from our New York sessions.
Greater Scrutiny, Greater Responsibility
One thing everyone working with data can agree on is that their sense of responsibility has steadily increased over recent years. This is due in large part to the greater scrutiny that governments, regulators, and companies themselves are placing on the development of AI in data science. At a panel on “The Future of AI in an Increasingly Regulated and Data Privacy-Conscious World”, Dataiku’s own Conor Jensen, RVP of AI Strategy, asked his two co-panelists, Ahman Khan and Jinkya Kulkarni, to weigh in on what this shift means for the industry.
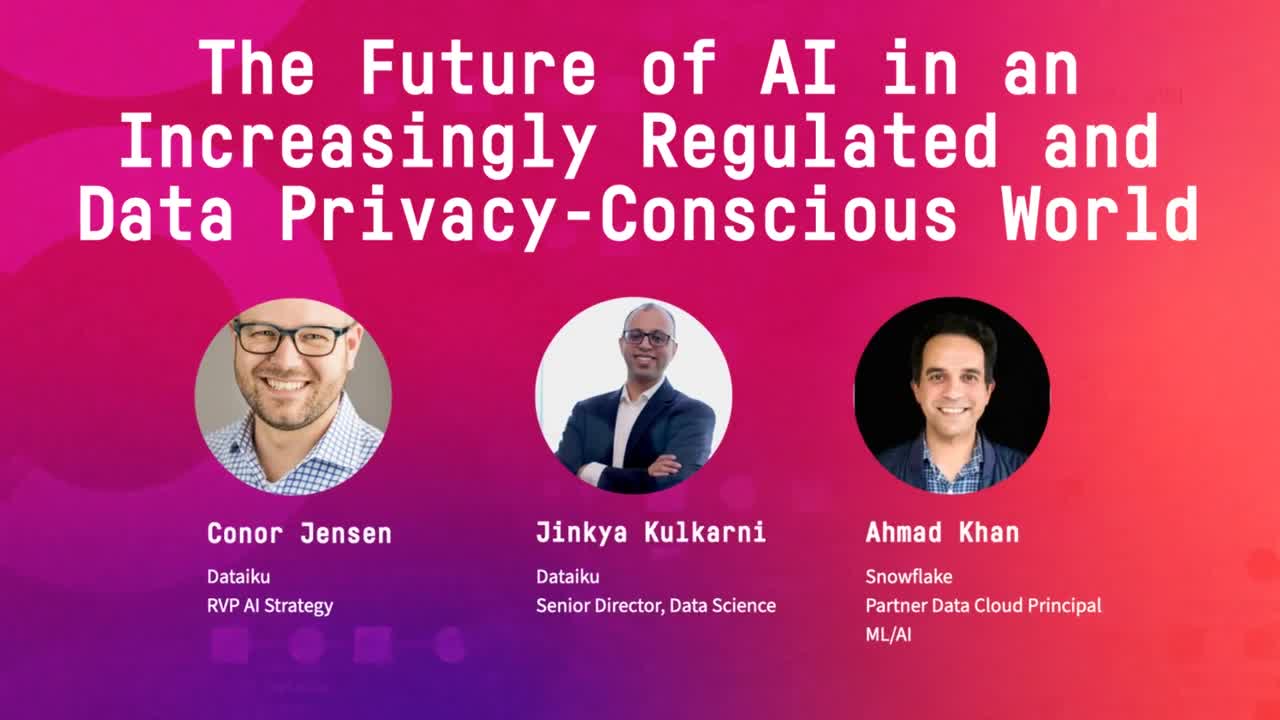
As Khan, Partner Cloud Principle ML/AI at Snowflake, explains, there are two sides to the regulatory coin. On the one hand you have data itself. In this case, the primary concerns revolve around privacy: who can gather, sell, and manipulate data, for example. These questions led to the development of data privacy regulations around the globe, including the Children’s Online Privacy Protection Act in the United States, and the General Data Protection Regulation in the European Union.
On the other side of the coin, though, is something equally important: model and algorithm regulation. As Khan points out, most data-driven companies have developed sophisticated responses to data privacy regulation, but they haven’t thought much about how to monitor and regulate their own models and algorithms. “Almost every data set is biased, as they say…. There’s bias in data and then there’s bias in models as well,” Khan said. “We’re just scratching the surface when it comes to the algorithm and the model side.”
The scant development of model and algorithm monitoring has been met with a correspondingly vague response from regulators, whose primary approach to handling cases of model mismanagement seems to involve ordering “algorithmic disgorgement” or the destruction of a company’s algorithms. It is incumbent upon both companies and regulators, Khan said, to have a clear understanding of how any given model makes decisions. That would enable interventions that are more nuanced than wholesale algorithmic dismantling.
It’s All About the Process
Another way to state Khan’s point is to say that companies need to have a firm grasp on their own data processes. This was echoed by Kulkarni, Senior Director of Data Science at Dataiku, who pointed out that, as companies strive to accomplish more sophisticated tasks using alternative data, they need to use increasingly sophisticated models to do so. But these new models, in turn, often find themselves suddenly unable to pass through the existing data processes. As a result, “You don't understand how the model makes the decision. You can't explain it,” Kulkarni said. And this is a problem.
“What's missing is not the tooling or the technology,” as Khan put it, but the processes. And this is also a problem on the consumption side, when, say, a data application is being used to drive business insights. “What are the metrics that you’re visualizing or monitoring? When a certain threshold is crossed, what ticket is generated?” Khan said, posing the questions that a process-conscious data consumer will ask. The pipeline that produced the outputs in question needs to be understood so as to avoid creating a black-box effect.
While agreeing with the centrality of processes, Kulkarni dissented slightly as to it being the be-all and end-all. “I think that process is a really good place to start. But to talk from a practitioner's perspective, the two things that I think are really essential are applying and iterating,” he said. Data teams need to build as they go, in other words. They need to develop processes, yes, but then they need to “tak[e] that process into application land where [they are] allowing the practitioners the chance to apply it, to iterate it, and quickly learn from it.”
Bracing for Change
The change to regulation is coming, especially in regard to algorithm and model monitoring. As Khan said, “there will be more and more regulation and oversight on the algorithm piece — the consent piece is already there.” And as regulators become more heavily involved in scrutinizing the models deployed by various companies, those companies will need to learn how to cleanse models of faulty predictions and insights without destroying them completely. Most importantly, they’ll need to learn how to communicate why these predictions are produced and in what cases.
Bracing for the change, moreover, means being not just lawful, but also responsible. Regulation will always be behind technology, as Kulkarni noted. But while it might be tempting to stay at the bleeding edge of what’s technically legal, it is not always ethical, and therefore responsible, to do so. “Do you really want to be on the front page of a national newspaper because…your algorithmic team messed up?”
The panelists also wondered whether and how Responsible AI might extend to consumers. Is it our responsibility to teach them how to look under the hood? Or is it theirs to learn? These were among the tough questions that Jensen put to the panel.
For Kulkarni’s part, an ideal near-future would involve a non-profit, neutral body whose “job is to help…create standards, create those training pads so that everyone is singing form the same song sheet, and everyone has the same reference.” Khan thought that the solution lay, at least for the moment, within. In data science, he pointed out, you often have three or four different personas responsible for building and testing an application, and you’ve got many others interacting with it downstream. The idea is to develop a way for each of these people to be mutually responsible: to know their duty and to know the duties of others. Ideally, Khan said, “you know exactly who is responsible for data quality and exactly who’s responsible for making sure the model is accurate.”
Whether the future more closely resembles Kulkarni's or Khan’s vision is impossible to predict. But what all the panelists could agree on is that “it’s going to happen organically,” per Khan. In what manner and with what speed any given company develops Responsible AI depends on the skillset and makeup of their data teams—and it will depend, too, on the products they use. “It takes a village to raise a child,” Kulkarni said at the panel’s close. “It’s going to take a village to raise AI as well.”
Governance for the Future
As Khan pointed out during the panel, the right product can make a good amount of the difference. When it comes to developing an ethos of mutual responsibility, for example, Dataiku can help data teams develop transparent, well-governed processes that allow companies to scale with both agility and control.
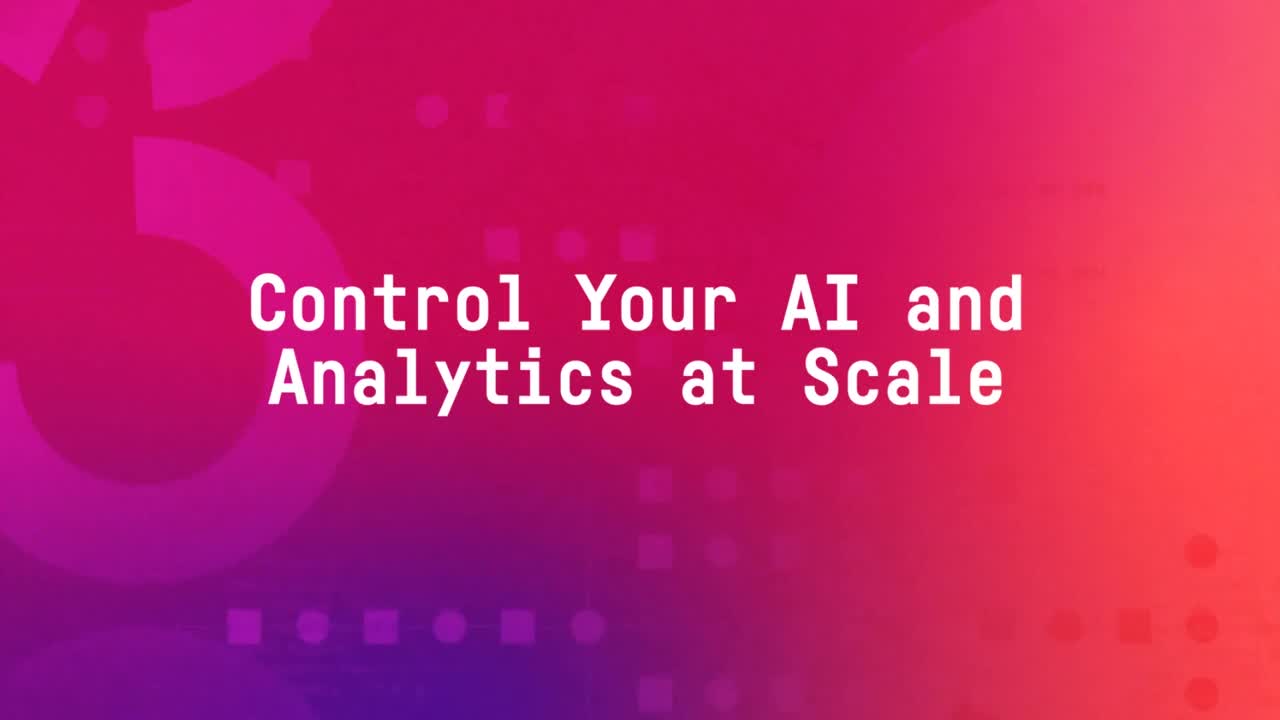
As Paul-Marie Carfantan, Solutions Consultant for AI Governance at Dataiku, explained in his talk on Day 1 of the conference, agility is almost never the problem for most companies. In the majority of cases, control is the pain point and, ultimately, the obstacle.
The reason control or AI Governance often take a backseat usually boils down to a combination of four factors:
- Lack of oversight: various stakeholders lack visibility onto the health and development of existing projects
- Lack of standards: project workflows, duration, and depth often depend entirely on each project owner and his or her skills and interests
- Time intensivity: Governing existing projects and models tends to be done manually (with, e.g., Excel) and thus slowly
- Low traceability: Decisions are not well-documented or easily accessible for reporting
Developing good governance, Carfantan said, is a little like building a house. It requires a steady process completed in stages. During the Grounding stage, the process is non-existent and the needs are not defined — you’re just getting started. During the framing stage, the process is informal and the needs are developing. And during the Trimming stage, when the house is built but the details need constant work, you have an established process and a well-defined set of needs.
To attain a heightened level of governance, companies need to find the right balance between the Business and AI roles, which oven prioritize agility, and the Control and Risk roles, which prioritize control. In fact, it’s not just about balance — it’s about mutual reinforcement. Each can learn from the other. As Carfantan put it, “Governance and Scale are not mutually exclusive. They can coexist together and really reinforce each other.”
Good Governance With Dataiku
Any good governance strategy will do three things:
- Centralize and prioritize by putting everything in one central place
- Explain and qualify by document everything, and keep everything clear to all stakeholders
- Deploy and monitor
Dataiku can help on all three counts, by enabling users to track the progress of AI projects in one centralized place and determine the governance levels required for each of them. By deciding what to govern and what not to govern, data workers can ensure they are developing Responsible AI practices while also gaining a lot of time via their discernment.
The software also allows you to document everything and define the business initiatives to which each project belongs. Users can easily ascertain a project’s scope and see exactly where and in what manner it will be deployed. And they can breakdown views according to everything from geography of deployment to whether a project is housed in HR or R&D. Dataiku Govern ensures that monitoring occurs before and after deployment, so that no project runs before its time, and none commits unnoticed errors or makes faulty predictions.
The Power of Self-Scrutiny
With Dataiku Govern, data teams can employ the kind of scrutiny normally reserved for regulators for themselves. By keeping projects central, documented, and monitored, they can remain responsible while also benefiting from the agility derived from time gained and communication simplified. And on top of that, they can maintain a clear understanding of their own process from start to finish, meaning it will never be difficult to keep regulators apprised of how, when, and with what effects a prediction was generated.