The other day, I was talking to my friend who runs a personal e-commerce site selling speciality items. He wanted to understand how machine learning technologies could help him manage his site better than he could by himself. Although I'm not into leather stuff, I was very happy to answer him.
I took for an example the contents of his site's "Leather Boots" page. In his eyes, deciding how to add content to this page was pretty obvious. As for what to put as the first item on the next page: the best-selling items, naturally.
Yes, but how do you define "best-selling"? The products that generate the most revenue in absolute terms? The products with the best conversion rate? Another, more advanced variant involves ranking products by multiplying their conversion rate by their gross margin. Indeed.
Behaviors Are More Complex Than Ratios
The way to model this problem using predictive analysis is the following:
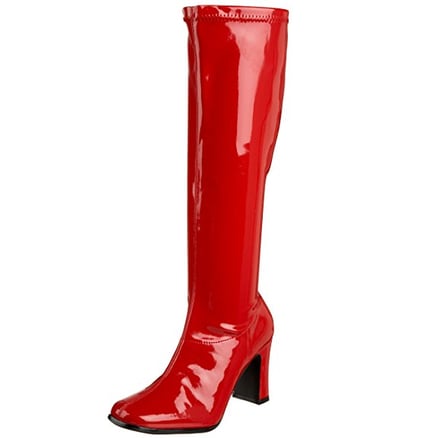
For a given user, at a fixed point in time 't', what is the product list 'p1,…pn' to display in such a way to maximize revenue?
Using the conversion rate times, a product's margin provides a perfectly reasonable method for estimating this probability. However, it also implies a certain number of assumptions.
-
The first assumption is that the conversion rate is more or less constant throughout a product's lifespan. Except that quite often, demand for a product will vary over its lifetime, depending on the season, sales promotions, or simply the length of time the product has been available online.
-
Another implicit assumption is that we chose each product independently. But that isn't necessarily always the case. For example, even if red boots regularly outsell black boots, it would be foolhardy to put only red boots on the landing page. Why? Because even if there is a strong red shoe contingent out there among your customer base, you need to include some black boots to satisfy the population that cares about black boots and black boots only.
-
A third assumption is that buying behavior doesn't change over time. But there are in fact many circumstances, like sales or an expected paycheck at the end of the month that influence buyer behavior, and the buyer's preference for more or less expensive articles.
-
A fourth assumption is that all buyers behave in the same way. In reality, their geographic location, their city, or their past buying behavior could allow you to predict which type or brand of boot to display, such as displaying cowboy boots for customers buying chaps, but not necessarily for other customers.
And then, off the top of my head, I began listing the implicit assumptions of the mathematical rule of three.
At this point, my friend interrupted me:
"But how much more could I earn using a more advanced technique?"
I replied with:
"I don't know, perhaps... 30%?"
I'll continue this explanation in another post.