Imagine: you have a global e-commerce company selling and shipping products all around the world. You would love to be able to predict which customers will bring the most value to your business so that you could target similar potential customers.
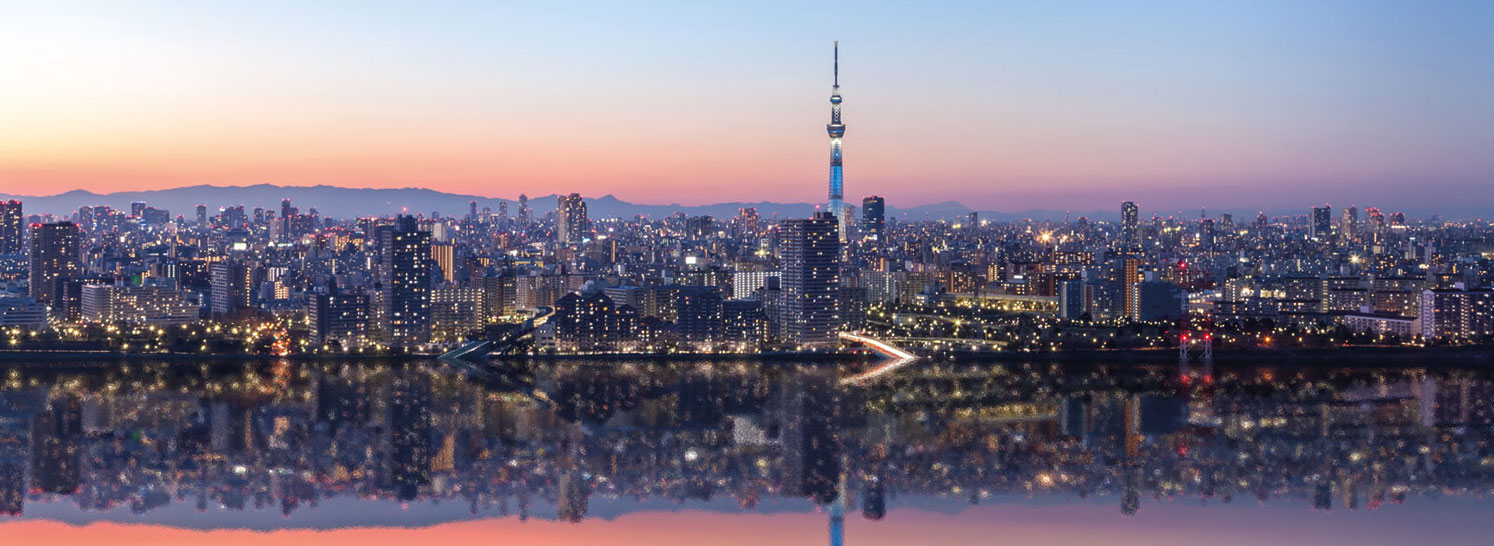
In our sample project gallery, we’ve created a project that lets you immerse yourself in this scenario. From data preparation to machine learning, you can see a predictive data project in action within the context of Dataiku. If you've never used Dataiku before, no worries — you can use it right in your browser without downloading anything.
First, Join All Your Data — Simply
In our example, you have two internal databases at your disposal. First, a customer relationship management (CRM) database records the value of each purchase made by each customer, along with information like their age, address, and gender. Next, you have a database of weblogs, which tracks how many pages are visited by each customer, as well as whether the specific customer has been targeted by a marketing campaign.
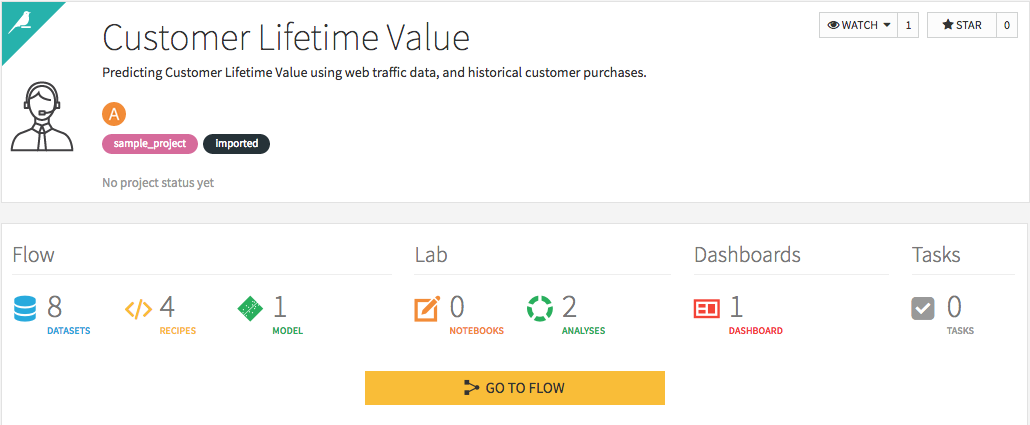
The project homepage - dive in and explore!
Additionally, you have a table with the per capita GDP of all countries in the world. Within Dataiku, it’s extremely easy to join this external table to your two internal databases — not a single line of code necessary!
Powerful Machine Learning Models for All
Now it’s time to build your predictive model. We’ll train a random forest model — a classic machine learning algorithm — to predict which customers will have the highest lifetime value. Once again, we don’t have to use any code in order to create this model in Dataiku.

Details of our predictive model — not bad, right?
Two things jump out: first, the model has an AUC of 0.892 — that’s pretty good! Secondly, the single most important variable turns out to be the country per capita GDP. It turns out that our external data source was pretty valuable!
Now we can actually put this model to use by “scoring” (applying predictions) to a new set of customers. The implications are clear: the marketing campaign doesn’t seem to do a whole lot, but customers from wealthy countries tend to spend a lot. So maybe it’s time to focus your marketing spend on some strategic countries!