We wondered why some companies successfully implement machine learning models into their operational system, and others don't.
So we asked! And from the results of this survey, we came up with a report of best practices for companies at different stages of data organization maturity. We wanted to take this one step further and analyze what big trends we could find in our survey results for the first quarter of 2017. Here are the top trends in the deployment of machine learning algorithms into production.
DATA QUALITY
50% of survey respondents agree that the biggest barrier to data deployment is data.
Data quality and pipeline development issues (and having the time available to work on data) are the number one issue. Access to data, data wrangling, and consistency of live data are different aspects of this issue.
IT CONTROLS PRODUCTION
50% do not have a specific data science production procedure.
Data production and processes is an IT-lead project (only 17% use PMML). The disconnect between data and IT teams can lead to recoding and longer design-to-production processes.
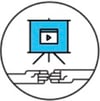
BUSINESS COLLABORATION
Only 33% of companies have close collaboration between business and data teams.
The main mode of communication on data projects is still PowerPoint or live dashboards (for 70% of respondents) rather than co-creation and co-monitoring of data projects.
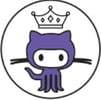
KING GIT
50% of companies use classic configuration management tools (like Git).
This is representative of an IT-led process with close attention to monitoring but doesn’t replace a rollback strategy dedicated to data projects running in production.
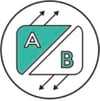
A/B TESTING IS THE RULE
76% report using A/B testing for model optimization.
And more than half of the respondents have built a dedicated framework to perform these tests rather than look into more complex and dynamic adaptive test systems.
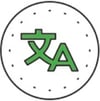
MULTIPLE LANGUAGES AS A NORM
80% of people have a polyglot development environment.
With different team members using different technologies to fine-tune, this allows for better data products. On the other hand, the skillset and technology discrepancies can complicate production processes.