By "efficient" data science team, I mean "data science that brings maximum productivity."
I've been reading a lot of data science articles in the past year or so, and one thing has struck me. I have read countless articles that focus on the best new models to win a Kaggle, the fastest data prep tools, the coolest projects, the impact of AI / blockchain / chatbots, etc.
I have, however, only read one article that gets real about how to build hardcore data science in practice (this one).
This is a problem because a majority of companies today report that their data science efforts are not bringing any value to their business.
The link between these two facts is simple: data science teams should be focusing on building projects that will be deployed into production and bring value to their business as well as the design phase: preparing data, analyzing it, and training models.
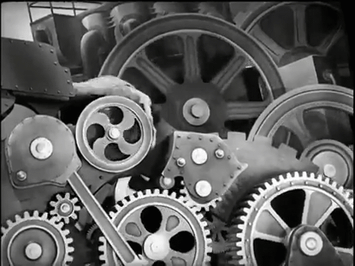
Efficient Data Science Teams Focus on More Than Insights- They Deploy in Production
Can't quite see the difference? Well, think of a data team analyzing a huge dataset of all of their users' actions to determine what their "wow" moment is and what product features create stickiness, for example. They explore the dataset, do some advanced clustering, and go to the product team to tell them what works (and doesn't). These are very valuable insights. However, at the end of the day, when the product team releases a new product based on those recommendations, they'll be the one credited for a reduction of churn.
That same team can then also use that same data to train a model that predicts what page a user needs to see at what moment to stick. They would then deploy it into production so it is integrated to the company's site and pushes content based on other users' previous actions. In this case, a reduction of churn would be directly related to the data team's actions.
This could sound like just a question of pride, but let's be honest for a second: in business these days ,any team has to be able to prove what they're bringing to the business to justify their budget.
6 Reasons Why Your Team May Not Be Production-Ready Yet
The reasons that data teams todays don't manage to deploy into production are not simple and straightforward, of course. But here are a few:
1. Too Much Data to Explore, Too Little Time
With the rise of awareness in business spheres that AI will make them more competitive, companies now have more sophisticated data storage and management. So, data scientists have access to that much more data that they never knew about. Before they can make anything of it, they need to spend time exploring it and understanding it.
2. A Focus on Machine Learning in Data Science Programs
Data scientist is not a job that has been around for a long time, so a lot of data scientists are fresh out of school. And what do they learn at school? Well, they learn to design data projects on Kaggle datasets. Real-time scoring technologies and other production- specific technologies are very recent, so they may not have even heard of them at school. Their studies just don't focus as much on getting them into production.
3. Production Is Technically Challenging
Deploying into production requires a lot of technical considerations from your IT department. You have to think of packaging for release, how to optimize models and keep making them better, and have roll-back and failover strategies in case something goes wrong. You also have to worry about monitoring, both technical and from business users, as well as how to audit the project or ensure IT environment consistency. And you have to worry about making all that scalable. And don't forget looking into real-time scoring or online learning. As I said, a lot of technical considerations.
4. Collaborating With IT Teams Is a Must
And speaking of the IT team, another reason production is hard is because it requires an extra level of collaboration. Projects that are developed by the data science team on extracts of data sometimes have to be completely recoded by the IT team! To make it more efficient, both teams have to work on the project together from the start so it can easily fit into the existing IT environment.
5. Risky Business
Deploying into production is much riskier than exploratory data science! If something fails and it's not on a server somewhere, it's live! So...
6. Everyone Needs to Have Your Back
Having data science models running in real time (or nearly real time) requires that the whole company is aligned and supporting the data science efforts. It requires involvement from the IT team before, during, and after to monitor drift and help with iterations on the project. It also requires that your business people participate in the project so it matches their needs and is usable by them on the long term.