ESG, SRI, impact investment: all of these names and acronyms point to the same reality, which is that the demand for responsible investment has moved from promise to fact. The need for long-term appreciation of risks beyond pure financial factors — even more so in the face of today’s uncertain health crisis — is pushing for accelerated demand from investors toward the full integration of Environment, Social, and Governance dimensions in the investment strategies run by asset managers.
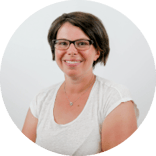
About the Author: Sophie is VP Strategy at Dataiku where she drives strategic projects and helps financial players on their path to Enterprise AI. She has 14 years of experience in the asset management industry and notably acted as COO for a multi-asset portfolio management division, conducting large scale IT, regulatory, and transformation projects, including active development of responsible investment.
“Our ambition is to better take into account environmental, social, and governance-related risks in investment decisions.” What could sound straightforward is turning into a true mind bender for all investment professionals who are confronted with pressure from regulators, NGOs, and clients to act and demonstrate.
At the same time, the markets are still very much trying to get a full grasp on the topic, struggling to put frameworks in place on subjects (not to mention public debate and regulations) that continuously evolve. On top of this uncertainty are strong local specificities plus a general lack of stakeholder alignment on the appropriate KPIs to track the different ESG components, and it’s easy to see why the topic is so complex.
But waiting for norms to emerge is not an option: asset managers who do not position themselves in a credible manner will simply fail to survive in a market already marked by increased competition, challenges of traditional active strategies by passives and alternatives, and continuous cost of regulation.
Out of the several ingredients needed to win the race for ESG, two stand out as essentials:
- Strong convictions, embedded throughout processes and built on…
- Distinctive ESG models capable of delivering strong ESG-adjusted performance and adapting to ongoing evolutions of the ESG landscape.
More and more, investment credos are beginning to evolve and assess the need for alternative data — there's a growing demand for investors to take ESG metrics into account in their investment processes in addition to the low-yield environment pushing for search of additional returns, and both of these are driving the push toward alternative data. To learn more about alternative data in financial markets, check out our comprehensive ebook.
It’s a Data Jungle Out There (& Getting Thicker by the Minute)
Asset managers who enter into the ESG space have to learn to navigate the world of external data providers with their respective frameworks, coverage, naming conventions, and types of data. To which they need to add all the material provided by brokers, research bodies, specialists, NGOs, and news feeds.
Asset managers who want to be serious about ESG more often than not end up juggling more than a dozen providers on top of their traditional financial data sources. And this jungle only gets thicker by the minute: as some large providers (such as MSCI and Sustainalytics) start emerging as industry standards and become commodities, asset managers are encouraged to combine these new must-haves with data issued by smaller organizations focusing on specific topics to have detailed impact tracking and preserve a differentiating edge.
To build their framework, asset managers will have to make choices, resting on the right selection of large providers, niche organizations, and public data, combined with internal insights and proprietary signals built from unstructured data. The capacity to blend, test, complete data coverage through machine learning, reverse engineer, and quickly review over time will be paramount to win the ESG race. So will be the capacity to enrich the set foundations with new data sources. For example, in an environment marked by new extreme weather hazards, can satellite images step in to assess emerging risks of exposure to flooding?
Turning the Switch to ESG: The Need for Collaboration
For a traditional portfolio manager, entering into the field of ESG starts with data. ESG scores aim at giving an overview of the ESG health of an issuer or asset, including controversy scores, CO2 emissions, or revenue exposure to sensitive activities such as coal and weapons production, etc. But asset managers can’t expect all their professionals to become ESG experts over the course of a few days (or even a few weeks or months).
Furthermore, ESG data should not be seen as just a simple addition to existing framework; it behaves fundamentally differently than traditional financial data, and more often than not, it demands full end-to-end rethinking of processes.
If the ambition is to manage portfolios with the objective of alignment with a 2° scenario, should this link to the exclusion of full sectors — and is that manageable from a liquidity and diversification standpoint? What are the appropriate indicators and how do they fit in with traditional financial indicators? Are their ways of developing proprietary signals to give forward views on stock or yield-curve evolution based on environmental performance?
Putting such frameworks in place requires bringing together ESG experts along with traditional portfolio managers, portfolio engineers, and operation process owners around the same environment to jointly build the right models. Keeping this topic a specialist one is a tempting route, but it will not produce the internal buy-in nor the models that can, in the long run, deliver true ESG risk-adjusted performance.
Sharing the Right ESG Impact View
The ultimate objective of responsible investment is to better hedge investors versus emerging ESG risks, aligning their investments with their own beliefs. This might mean covering desire to disinvest from controversial activities such as coal production, tar sands, weaponry, palm oil, or to actively tune exposure toward social wellbeing, green activities development, gender balance, etc.
ESG topics don’t stop with asset classes. A growing demand is emerging for cross-asset class views with consolidated perspectives on risks and exposures (beyond traditional ways of reporting and managing assets). To develop this type of ESG fiduciary and advisory service, asset managers will need to build new models as well as demonstrate agility to preserve their operational core foundations while building views and advice adapted to the specific demands of their clients.
Working with traditional frameworks will not solve this complex equation. New data types will need to play a role, along with new types of stress tests and models to help clients make the appropriate decisions. Fully preparing all business lines to foster these models and demonstrating proactive advice to clients using resulting learnings will be among key ingredients to emerge as true ESG leaders.
The Bottom Line
Most asset managers — not to mention investors — are only starting their journey on ESG. The topic remains a true shape-shifter, supported by strong local specificities, which makes it all the more difficult to capture. That makes it even more important to recognize the inherent complexity of the topic and the need to root answers in:
- Solid data and modeling foundations, combined with...
- Collaboration across all experts