A few weeks ago, OpenAI unveiled GPT-4o, its latest and most advanced transformer-based foundational model. The “o” in GPT-4o stands for "omni" (Latin for “every”), reflecting its ability to handle prompts that mix text, audio, images, and video. This powerful multimodal capability has captivated the AI community and set a new standard for generative and conversational AI experiences.
But, alas, in today’s fast-paced tech landscape, attention spans are fickle and it’s hard to maintain leaderboard status and media dominance for long. Mere days after OpenAI impressed us with its GPT-4o model, Google followed up with a video showing off its Gemini 1.5 Pro model using live video and spoken prompts to provide information on a Pixel smartphone. Remember the buzz about Mistral AI’s Mixtral 8x7B in January, followed by Mixtral 8x22B in April? How about the debut of Anthropic’s Claude 3 in March and Meta’s Llama 3 splash in April?
Each of these groundbreaking models made waves within the last half year alone. With each month that passes, it seems like a new model advances the leading edge and steals the spotlight. As a data scientist or enterprise data leader, no one would blame you for suffering from analysis-paralysis: Should you build your GenAI-powered application on top of today’s best model or hold out just a little longer until a new one emerges that’s more accurate, powerful, or better suited for your use case?
Ride Now or Wait?
Put differently (and no doubt colored by anticipation of my upcoming trip to the beach), here’s the question at hand: Everyone knows they need to capitalize on GenAI momentum or risk being left behind, but with the relentless waves of model innovation coming so thick and fast, how can you be sure you’re picking the right wave to surf? Should you just be patient and wait for things to settle?
The short answer is: Don’t wait. We are in the midst of an epic model release battle between major AI players, with new entrants emerging all the time. To date, there are more than 125 commercial LLMs in the model landscape, with an astonishing 120% increase in models released from 2022 to 2023. And that’s not even taking into account the hundreds of thousands of open-source models available in HuggingFace! Models are becoming more performant, specialized, and multimodal.
But you can’t sit on your board watching the horizon indefinitely. With 88% of executives already investing in GenAI (according to a MIT Technology Review Insights report with Databricks), waiting for the perfect wave is a strategy for competitive disadvantage at best, and obsolescence at worst.
There will be two kinds of companies at the end of this decade...Those that are fully utilizing AI, and those that are out of business.
— Peter H. Diamandis, Founder and Chairman of the X Prize Foundation, Cofounder and Executive Chairman of Singularity University
The Risks of Over-Reliance on a Single Technology
That said, it’s also risky to rely too heavily on a single model. Consider the well-known case study of IBM Watson in Healthcare: IBM centered its commercial healthcare applications around Watson’s AI model without integrating or cross-validating results with other models or data sources. When reports emerged that Watson sometimes provided inaccurate or unsafe treatment recommendations, market trust eroded, and IBM struggled to recover from the sunk development costs and technical debt.
Just as it's unwise to rely too heavily on a single model — even one as impressive as OpenAI’s GPT-4o — it's equally risky to anchor your strategy to a single provider. Despite OpenAI's status as a leader in AI innovation, recent scrutiny over its governance and leadership has raised concerns among investors and enterprise adopters. To navigate this fast-changing landscape and reduce operational risk, avoiding vendor lock-in is crucial to ensure you can seamlessly switch between AI services as needed, whether due to technological advancements or concerns about security, ethics, or organizational stability.
Surfing Smart With the LLM Mesh
The good news is that there’s a balanced approach; you can build solutions now while preparing for future changes. With Dataiku’s LLM Mesh, you can develop AI applications using the best models available today and still maintain the flexibility to switch to more suitable providers or models as they emerge.
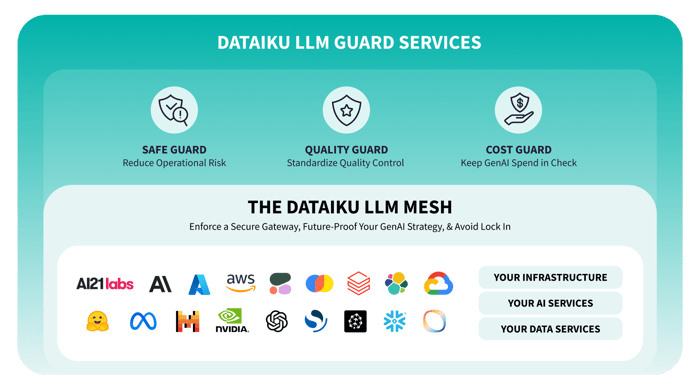
Flexible AI Development
The LLM Mesh acts as an abstraction layer between LLM providers and end-user applications. It allows for rapid “wave hopping” between different LLMs during the development and iteration phase, so data scientists and AI engineers can evaluate which models provide the most accurate results for their specific use case, estimate costs, and make informed trade-offs between performance and price.
Simplified Integration and Management
In keeping with Dataiku’s core values of infrastructure agnosticism and platform interoperability, the LLM Mesh abstracts away the complexities of backend connections and API requirements, making it simple to transition from one model to another, even after the project is in production. This means you can keep the pipeline and user application stable while adapting and evolving the backend over time to accommodate new advancements — no need to kick out and paddle back to the lineup to catch a new wave!
In summary, the LLM Mesh offers a flexible, future-proof way to build AI applications. With Dataiku, you can confidently ride the leading edge of today’s technologies while staying positioned for the next set, ensuring continuous innovation and competitive advantage.