Data teams are increasingly exploring AI agents to automate complex workflows, deliver personalized insights, and augment decision-making processes. In a recent webinar, Nanette George from Dataiku's Product Marketing team and Daniel Ionita, a Dataiku Senior Solutions Engineer, demonstrated the practical process of building, testing, and debugging AI agents in Dataiku. This session provided valuable insights for data professionals looking to implement agentic capabilities within their organizations.
Here’s a breakdown of the key learnings from the webinar and how Dataiku simplifies building robust AI agents with precision debugging capabilities.
Understanding AI Agents: Beyond Simple Chatbots
Before diving into the technical implementation, Nanette established a clear definition of AI agents: an LLM-powered system designed to achieve objectives across multiple steps and generally with a concept of going beyond just answering a question.
While chatbots can answer questions from their training data, AI agents take additional steps and perform actions. For example, a chatbot might answer questions about company expense policies. An agent could review receipts, check them against policies, flag issues, route approvals, update systems, and send email notifications — all autonomously.
During the webinar, Nanette conducted a poll to understand where attendees stood in their AI agent journey. The results were revealing, with 86% of the 168 attendees who responded indicating their organizations were building agents, plan to do so, or have agents in production:
- 48% poll respondents weren't yet building agents but had plans to create them
- 28% were building agents
- 10% had successfully deployed agents in production
- 14% indicated they weren't planning to create agents
Building a Market Research Agent in Dataiku
Daniel demonstrated how to build a practical, real-world agent using Dataiku's visual interface. The use case focused on a market research agent that could:
- Look up topics of interest for specific companies from a database, dataset or knowledge bank
- Search the web for the latest news on those topics
- Compile findings and send an email summary to stakeholders
Daniel began the demo with importing and preparing a simple dataset containing companies and their associated topics of interest. He used the Dataiku platform's natural language interface to split the topic column on commas, demonstrating how data preparation capabilities are integrated throughout the platform.
Using Agent Tools in Dataiku
Using the Dataiku platform's toolbox approach, Daniel could select tools for the agent that avoided the need to use coding. In the Dataiku platform, there are pre-built agentic tools and tools that are created by other users and made available for use in the platform. Daniel chose three tools for his agent:
- Look up a record in a dataset: Configured to retrieve relevant topics for a given company name from the prepared dataset.
- Search the web: Set up to search Google for news articles about specific companies and topics.
- Send a message: Created to compile findings and deliver them to stakeholders.
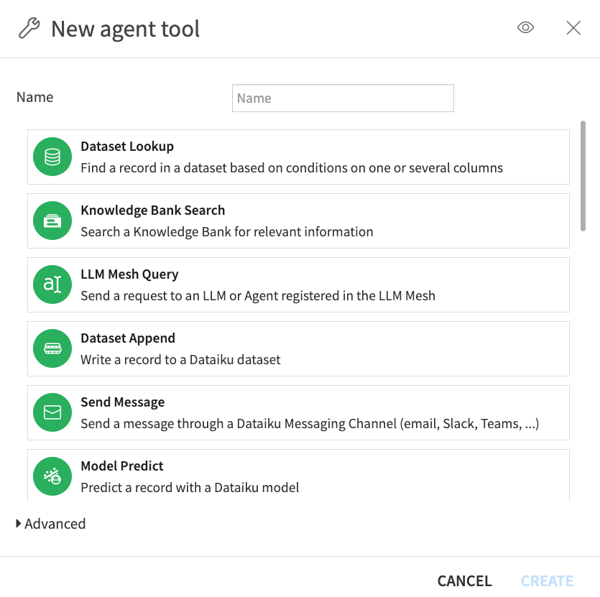
In the Dataiku platform, there are pre-built tools for working with agents and tools that are created by other users and made available for use in the platform.
Instructing and Testing the Agent
After configuring the tools, Daniel defined the agent's instructions using this natural language prompt:
"You're an expert at summarizing the latest news using the search web tool and helping a user keep up with the latest advancements. Given a company name, use the get topic tool to find the relevant topics for the given company, then create three bullet points with what you find in the news. Provide a link so the user can visit the source articles. Once you gather those bullet points, use the send email tool to inform someone about the three bullet points you found."
The agent seamlessly executed this workflow end-to-end, retrieving topics associated with Anthropic, then searching for relevant news, compiling the findings, and sending a well-formatted email with informative bullet points and source links.
Building a User-Friendly Interface
To make the agent accessible to non-technical users, Daniel demonstrated how to create a simple chat interface using Dataiku's web application capabilities. This allows users to interact conversationally with the agent, asking follow-up questions after receiving email summaries.
Daniel emphasized that this approach makes agents more accessible: "The advantage of adding a chat interface on top of this is that users could continue that conversation. Their emails could still be sent, but then they could ask why they should use this over something else."
Improving Agent Performance
Daniel continued his work on the agent by showcasing how to debug the agent he created. He explained how agents built with Dataiku are inherently easier to debug, due to the platform’s:
- Tool-based architecture: Each component can be tested independently by breaking complex workflows into discrete tools.
- Conversation history tracking: Saving conversations in a SQL database for performance analysis.
- Transparent execution: Trace Explorer makes it easy to see which tools the agent chooses to use and when.
Daniel highlighted that Dataiku's approach reduces the hallucination risks often associated with LLMs. “Adding tools reduces some of that hallucination, because instead of asking it to answer from its own knowledge, we showed you how to do a dataset lookup, for example,” he said. “These tools are predefined steps that you can test independently from the model."
Key Takeaways for Data Teams
As data teams begin exploring how to incorporate AI agents into their workflows, it's essential to have a clear strategy for implementation, testing, and maintenance. The webinar offered practical guidance for data professionals at every stage of their agent journey, from those just beginning to explore the possibilities to teams ready to scale their agent deployments.
Daniel emphasized that successful agent implementation requires thoughtful planning and continuous refinement. He shared these insights for data professionals looking to implement AI agents in their organizations:
- Start simple: Begin with clearly defined use cases where agents can add immediate value.
- Leverage visual tools: Dataiku's no-code approach allows rapid prototyping and iteration without extensive development resources.
- Test components independently: The tool-based approach allows for precise debugging of each agent component.
- Document agent capabilities: Clear documentation helps users understand what each agent can do and sets appropriate expectations.
- Implement evaluation: Use Dataiku's evaluation capabilities to track performance over time and iterate on improvements.
Why Build AI Agents With Dataiku?
While other platforms and frameworks offer ways to build AI agents, Dataiku provides distinct advantages that make it particularly well-suited for enterprise-scale agent development and deployment. The platform's integrated approach combines the flexibility needed by technical users with the accessibility required by business stakeholders, all within a governance framework that maintains control and visibility.
Throughout the webinar, Daniel demonstrated how a unified environment streamlines the entire agent lifecycle, from data preparation to deployment and monitoring, without sacrificing power or customizability. The Dataiku platform provides these capabilities to create a comprehensive environment for agent development:
- Single place to manage agents: Agent Connect provides a single interface for organizations to manage multiple specialized agents
- Advanced debugging: Trace Explorer, with tracing and optimization capabilities
- Cost and quality controls: Capabilities to monitor and control LLM usage costs and ensure reliable AI by automating evaluation and monitoring, all with the Dataiku LLM Mesh
The Road Ahead
For data teams already building or planning to implement AI agents, Daniel emphasized the importance of documentation, testing, and continuous evaluation:
"This is a very simple example, but with these components, you can build all sorts of agents that will solve problems you care about. Given that it's a data project, you could take it further and apply evaluations, you could perhaps apply some automation so people get their emails whenever they need to get them, really giving you the flexibility to enhance and add efficiency to your processes."
As organizations continue to explore the potential of AI agents, Dataiku's integrated approach provides a robust foundation for building, debugging, and scaling these capabilities across the enterprise.