Insurance is one of the many industries that has been tremendously shaken by the events of 2020. These unprecedented happenings are significantly reshaping the insurance market, pushing insurers to review their approach to numerous sectors and risks.
This market shock should not overshadow the longer trends at play which are sustainably transforming the insurance business, across all business lines (P&C, life, savings, and commercial): longevity, health conditions, climate change, and the combined impact of stronger competition and demand from increased customer centricity from the market.
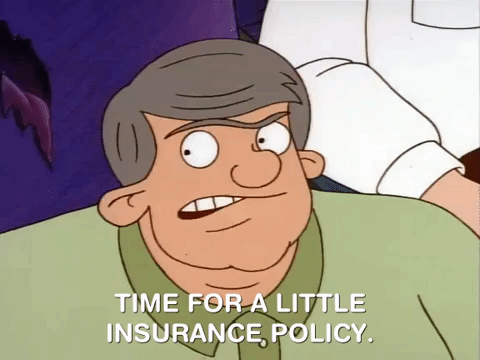
How Important Is AI to the Future of Insurance Organizations?
In order to strive in this context, insurance companies need to consider their level of AI adoption and maturity to assure they have the necessary resources and abilities to future proof their business.
In September 2020, Dataiku sponsored a benchmarking study of over 1,200 data executives on AI best practices, investment plans, and performance metrics.
When asked, 63% of insurance companies said AI is either considerably or very important.”
The global pandemic has made most evident that accelerated usage of data and enhanced capacity to predict impact and adapt are keys to success. The first step, however, is the democratization of access to data. By putting data in the hands of the many and breaking traditional siloes, companies gain agility in modeling and roll out. Through this type of collaboration and inclusivity, creativity and innovation initiatives are expanded to all individuals, thus bringing in diverse points of view. Only together can teams find solutions to their challenges.
Why AI Is Important for Commercial Insurance
These last few months have demonstrated how quickly traditional perspectives on industries can shift. This is why rapidity in model revisiting and roll out, enhanced by data democratization, is crucial today.
Another necessary skill is that of injecting alternative data in models. With end retailers going on and off activity on very short notice, are entire sectors to be banned from insurance, or can alternative models with adjusted pricing be offered to preserve support to the market? Insurance companies need AI to install multi-factor analysis and models to build a full picture perspective on risks and provide the best adjusted pricing.
Why AI Is Important for Retail Insurance
Having a tailored approach to individual risks, shifting from product- to customer-centric perspectives and developing end-to-end customer experiences to accompany customers through every stage of their journey is a priority in retail insurance. This can only be done accurately with collaboration between multiple teams and the support of appropriate data access, testing and roll-out, and a short revisiting cycle to build risk-adjusted brand preference.
Challenges and Lessons Learned Implementing AI in Insurance
It always helps to know the types of challenges that might arise on your Enterprise AI journey. The ESI Thought Lab survey examined the biggest challenges with regard to AI adoption through three categories: financial and regulatory, organizational/attitudinal, and technological. The top challenges in each category for the insurance industry were as follows:
- 53% of participants listed “regulatory constraints” as their top financial/regulatory challenge
- 51% of participants listed “challenges with implementation and project management” as their top organizational/attitudinal challenge
- 44% of participants listed “concerns about cybersecurity/data privacy” as their top technological challenge
Based off of these challenges, participants were able to offer what they believed to be the most
important lessons learned in implementing advanced AI within their organization:
While the specific approach will change from one company to another, integrating these learnings in Enterprise AI strategies will prove essential in successful scaling.
Conclusion
The potential scope of AI use cases is extremely broad, across all business lines and activities. The ultimate key to successful scaling starts with proving impact to build trust, and generate the right momentum to support a true AI-powered company transformation.