Data science teams are not that different from other teams within a company. So why do 85% of their projects fail?A quick Google search of the question “Why do data science project fail” makes two things immediately clear:
- A lot of people are having to deal with this problem.
- The reasons listed are generally the ones you’d expect of any failing team within a company — goals are improperly set, and communication between teams is not great.
The question then becomes a bit of a philosophical one — what is it about data science teams that make them so prone to failure? Executives have been able to deal with equally opaque financial models and marketing campaigns forever. But a Gartner poll puts a nearly 85% failure rate on most data projects — indicating that the issue must go deeper than simple confusion.
We'll explore some of the high-level reasons here, but for a closer look (as well as suggestions for resolution), check out the ebook 6 Key Challenges to Building a Successful Data Team.
Communication Really is Key
First and foremost, poor communication is a significant source of data project failure. Although this was touched on in a previous post, it really is an essential feature of any data project. If project intentions are improperly aligned with the goals of executives, then there is no hope of properly executing the project.
Diving a bit deeper into this, however, the idea of expectation versus reality has far-reaching implications for data teams. “Big data” has been one of the biggest buzzwords of the 2010s — and executives have unquestionably taken notice. Mismanagement of data projects might not only come from improper alignment of expectation, but rather from a misuse of data science as something to do simply so as not to be left behind.
Companies often employ big data solutions without understanding how the data teams can (or should) best be used.
Growth, But at What Cost?
While we mentioned before that Glassdoor ranked the data scientist as the best job in the United States, it is worth noting the immense growth that has taken place in data-related industries over the last few years. LinkedIn released a study that indicated nearly 10x growth in the number of machine learning jobs and 6.5x growth in the number of data scientist jobs between 2012 and 2017.
The process of hiring new employees and assembling teams is one of the first hurdles that must be jumped through. While it may be tempting to hire only PhDs in data, doing so limits the ability of your team to leverage complementary skill sets and unique perspectives. While data science is seen as this monolithic function of an organization, it is should be composed of people with a variety of capabilities and expertise.
Technological Woes
Another significant pain point for data projects is a misuse of available technology for the project at hand. Hadoop is useful only in certain circumstances, determined by the amount of data being stored, the types of data structures, and the intended use of the data. Therefore, you’d be doing yourself a disservice if you were to use it in a situation where an SQL BI tool or something of that sort would be more effective. The same could be said about programming languages and other data infrastructure decisions.
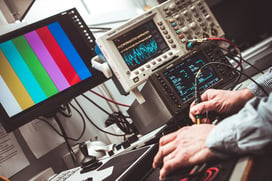
Additionally, many data teams begin to form models having participated in the cardinal sin of data analytics - using unclean data. Kaggle recently conducted a poll where nearly half of respondents said that a significant barrier faced at work was dirty data. Models trained using dirty data cannot provide meaningful insights — and so the improper cleaning of data is an almost sure-fire source of a failed data project.
All Roads Lead to O16n
In the end, all of these problems can be encapsulated by a single idea — too often data teams are formed and asked to carry out tasks without having an end goal of operationalization (o16n) in mind — and make no mistake: bringing data projects into operation is essential. If you are analyzing data and making models simply for the sake of making them, no one will ever be able to get real use out of them.
Clearly, data science will only grow more attractive to investors. That same LinkedIn article projects 250,000 available data scientist jobs by 2024. It is up to the hiring companies to make sure that they are not setting up their teams to fail.