Earlier this month, Kurt Muehmel was joined by guest speaker Michele Goetz from Forrester to discuss the topic of data quality in the age of Generative AI. If you want to catch the replay, you can find it here (or jump to the full video below), but we’ll also give a TL;DR recap of the big takeaways:
There Are New Challenges With Data Quality in the Age of Generative AI
It’s well known that bad data quality can have an impact on AI trust. In a September 2023 AI Pulse Survey by Forrester, 85% of AI decision-makers believed that internal data is high quality and ready for use in AI applications, however, 56% don’t actually trust the information provided by Generative AI. So, why the disconnect between belief and reality? There are three main issues when it comes to data quality with Generative AI:
- Generative AI incorrectly corrects: Similar to autocorrect changing the meaning of a word unintentionally, Generative AI might incorrectly infer meaning and deliver results that are inaccurate.
- Generative AI has imposter syndrome: At the same time, Generative AI models output what they “think” the correct answer should be but are resistant to correction or seeing fault in results.
- Generative AI hallucinates, creating outputs that seem accurate at first glance but, upon deeper scrutiny, are not accurate or miss the intended context or meaning.
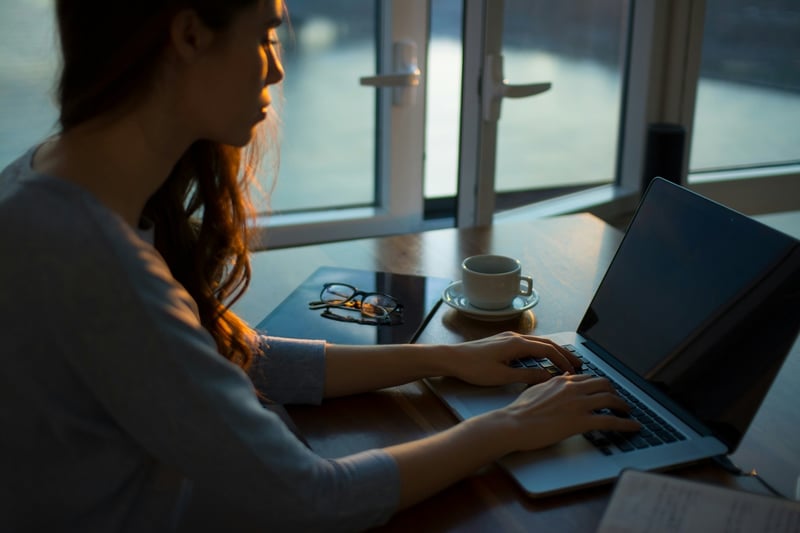
Good Data Quality Is Needed Throughout the Process of Prompt/Response
In order to ensure high data quality for Generative AI, it’s important to incorporate data observability, as systems shift to request: response. When creating prompts, systems and checks should be in place to ensure prompts are secure and valid. For example, determining when personal information should be obfuscated or certain prompts containing confidential information be rejected outright prior to being sent to an LLM.
Generative AI is grounded on semantics and ontologies that frame data understanding. Interpretation then turns into metadata. If metadata is created incorrectly or assigned to information incorrectly, discoverability of right and relevant data will in turn be incorrect.
And so, it’s important to also have visibility into the system assigning that metadata prior to getting to the LLM. While RAG addresses some concerns by drawing from known and trusted data sources, you still have to know where the data is coming from. When you move to a production environment, data comes from a multitude of locations.
A Generative AI system isn’t just information in a logical format, it’s a semantic system that lives off an understanding of ontologies. And so, you have to think about it more holistically to understand not only what types of info need to come into the system, but also ensure what comes out of the system is in the language of the business and readily understood and recognizable.
Addressing Data Quality in Generative AI
To address these challenges head-on, we need to steward our data differently and change the ways in which it is governed:
- Where you govern is important because it helps you understand data flows but also what additional context is needed for end users. Ensure you are governing where Generative AI is providing outputs, whether that might be a business application or at the edge.
- What you govern should include not only structured and unstructured data, but also metadata and concepts along with Inputs and outputs.
- How you govern should be focused on continuous curation, federated governance at the correct endpoints (i.e., within a vector database, or within a mobile application), and policy linking throughout the Generative AI process to ensure consistency.
- Who will govern should be distributed across the company, from business domains which should be owned by a business SME, to the logical domain which supports a database of critical data and is owned by the data management SME, to the physical domain and location of critical data which is owned by the database admin SME.
Ultimately, companies should:
- Expand data governance to improve AI Governance.
- Catalog data to set standards, controls, and workflows so that you’re orienting information toward what you can trust.
- Use Generative AI to improve data.
- Connect data security and privacy to data quality and trusted AI.
- Manage data as a product to align with the Generative AI system.
- Don’t just get AI literate, get data literate!