AI adoption requires a lot of cross-team engagement and collaboration, but the Return on Investment (ROI) is clear; according to PwC, AI has the potential to contribute $15.7 trillion to the global economy by 2030.
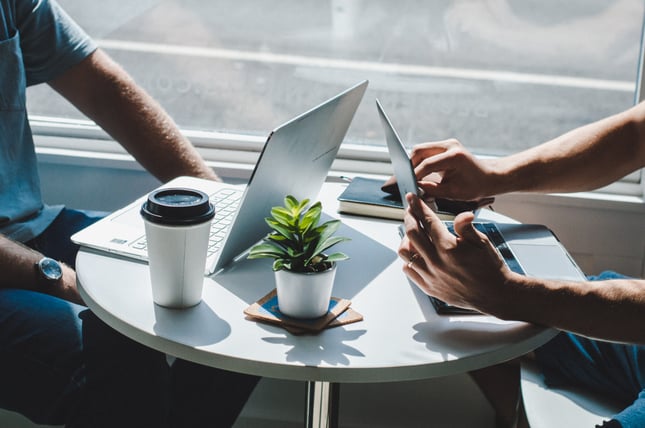
Once an organization decides to take the plunge, however, there are several potential hazards that could obstruct their realization of this value.
The Challenges
Dataiku has supported many organizations on their path to sustainable, Enterprise AI, and found that a few key obstacles can often impede successful AI adoption:
1. Skill Gap
A lack of necessary skills and expertise can often be a blocker in the early stages of AI adoption. Without a robust data or AI team in place, it's challenging to know how to fill these gaps.
Solution: Through investment in education and data democratization, analysts can be activated as citizen data scientists. Business employees already possess robust industry expertise, which means their targeted AI adoption will align with greater objectives. Through the use of robust tooling and AutoML, analysts and business users can expand their expertise and help fill skill gaps.
2. Hiring Talent
There are certain aspects of AI adoption that require data scientists' technical expertise. Scaling a data team is crucial to building the best models for an organization’s unique business concerns. However, looking for a "data unicorn," who possesses all the skills an organization needs, is an impossible (and expensive) task.
Solution: There is no universally perfect data talent, but there is talent out there that is perfect for each organization. Instead of looking for the data scientist that can do it all, focus on isolating the skills needed and finding the data talent that has some of these skills. This way, an organization can build out a data team and scale sustainably, while fostering collaboration and skill-exchange. This has the added benefit of encouraging an open data culture and improving retention of existing talent.
3. Rocky Foundations
If the technical infrastructure can’t support the necessary quantity of data or processing the organization requires, it will be unable to succeed no matter how well-designed the model is.
Solution: Intention and care need to go into data architecture design, that can be stable enough to support users’ needs, while also agile enough to scale as the organization grows. Calculating the ROI of architectural upgrades is challenging, but when data teams work with R&D to determine feasible ideals, and continue to improve as AI becomes more integrated into the organization, this should no longer be an impediment.
4. Data Collection
Machine learning models often benefit from new and innovative data sources, but a dearth of quality collected data can starve them. Without access to quality data (or the necessary data at all), models will be unable to generate precise insights.
Solution: This goes hand-in-hand with architectural improvements; by constructing storage and pathways to support quality data collection, and providing access to the tools necessary to process the data, data teams will be able to capitalize on an investment in data (over 40% of surveyed data practitioners find data cleaning and wrangling to be the most difficult and time-consuming part of the process).
5. Clarity of Vision
Without a clear AI strategy and goals, organizations often miss the most valuable places to start incorporating AI, which ultimately leads to diminished value and trust that an investment in AI is the right move. While the push for automation and AI is often a grassroots endeavor, without leadership’s direction, the momentum may be misguided.
Solution: When leadership really listens to data and analytics teams, they open up communication pathways and learn where AI can really drive measurable value. But it is leadership's responsibility to ensure that AI adoption aligns with an organization's overarching business objectives. Making sure that AI strategy has roots going from the top-down and the bottom-up will result in a more robust vision.
6. Defined Value
While many organizations have delivered upon the promise of AI, it is challenging to predict how it can provide concrete value to an individual organization. Uncertainty surrounding ROI expectations, and subsequent calculations on projects that can incorporate many teams and verticals, make value attribution a challenge.
Solution: Early syncs and communication on key ROI considerations across teams and verticals, followed by evaluations and adjustments, is the best way for teams to capture the complexities of ROI, and agree on the calculations. Again, communication and collaboration are key.
7. Silos and Segmentation
AI drives value best when data has an end-to-end path from collection to analysis, to insights and feedback loops. Organizational silos restrict these thoroughfares and prevent a rapid response to the insights AI provides.
Solution: An organizational overhaul can’t happen overnight; instead, focus on ways to help teams collaborate to operationalize data projects. Building on these foundations can improve the usefulness and accuracy of AI projects.
Avoid the Pitfalls
While AI Adoption reveals challenges and areas for organizational growth, the potential gains outweigh the costs. When AI is collaborative and aligned with business objectives, it supports an open data-driven culture and improved efficiency or user value. Take the plunge and see how much value inclusive AI can bring.